1. Introduction
The Pandas library, an integral part of the Python data analysis ecosystem, offers robust data structures and functions needed to manipulate structured data. Data analysis is a critical process of inspecting, cleansing, transforming, and modeling data to discover useful information, draw conclusions, and support decision-making. The Iris dataset, a well-known multivariate dataset introduced by Ronald Fisher, serves as an excellent starting point for beginners in data analysis.
1.1 Codewords To Remember the Data Analysis Process
S L U E V U M P I I
2. Setting Up The Environment
To begin with the data analysis, we first need to install and import the necessary libraries. The Pandas library can be installed using pip:
pip install pandas
Once installed, we can import the library along with other necessary libraries like numpy and matplotlib:
import pandas as pd
import numpy as np
import matplotlib.pyplot as plt
3. Loading the Iris Dataset
The Iris dataset consists of 150 records with five attributes – sepal length, sepal width, petal length, petal width, and class (Iris Setosa, Iris Versicolour, Iris Virginica). We can load the dataset directly from the seaborn library or from a CSV file using Pandas:
from sklearn.datasets import load_iris
iris = load_iris()
df = pd.DataFrame(iris.data, columns=iris.feature_names)
df['target'] = iris.target
df['target'] = df['target'].map({0: 'Setosa', 1: 'Versicolour', 2: 'Virginica'})
4. Understanding the Dataset Structure
Before diving into data analysis, it’s crucial to understand the dataset’s structure, including its dimensions and data types:
print("Dataset Dimensions: ", df.shape) print("\nColumn Names:\n", df.columns) print("\nData Types:\n", df.dtypes)
5. Exploratory Data Analysis (EDA)
EDA involves analyzing and investigating the dataset to discover patterns, anomalies, or relationships. We can start by calculating the statistical summary and checking for missing values:
print("Statistical Summary:\n", df.describe())
print("\nChecking for Missing Values:\n", df.isnull().sum())
6. Visualizing the Iris Dataset
Data visualization is a powerful tool for understanding and interpreting data. We can use Matplotlib and Seaborn libraries to create visualizations like histograms, box plots, and scatter plots:
import seaborn as sns
sns.pairplot(df, hue='target')
plt.show()
7. Univariate Analysis
Univariate analysis involves the study of individual features. We can analyze the distribution of individual features using histograms and detect outliers using box plots:
df.hist(edgecolor='black', linewidth=1.2)
plt.show()
sns.boxplot(data=df)
plt.show()
8. Multivariate Analysis
Multivariate analysis involves the study of relationships between multiple features. We can calculate the correlation between features using the correlation matrix and visualize relationships using scatter plots:
print("Correlation Matrix:\n", df.corr())
sns.scatterplot(x='sepal length (cm)', y='petal length (cm)', hue='target', data=df)
plt.show()
9. Preparing the Dataset for Machine Learning
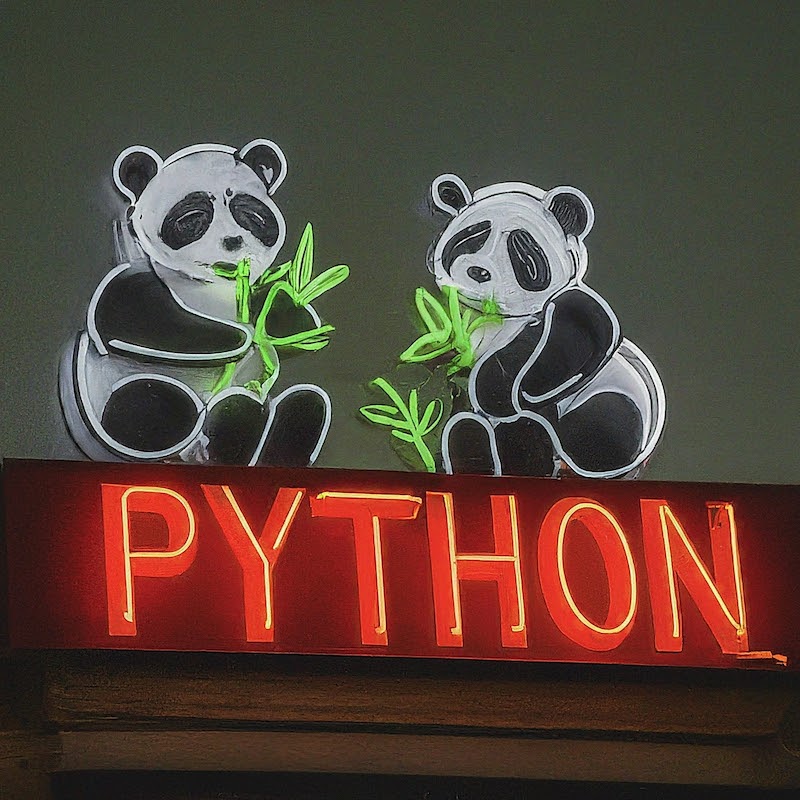
Before implementing machine learning algorithms, we need to prepare the dataset by scaling the features and splitting it into training and testing sets:
from sklearn.model_selection import train_test_split
from sklearn.preprocessing import StandardScaler
X = df.drop('target', axis=1)
y = df['target']
X_train, X_test, y_train, y_test = train_test_split(X, y, test_size=0.2, random_state=42)
scaler = StandardScaler()
X_train = scaler.fit_transform(X_train)
X_test = scaler.transform(X_test)
10. Implementing Machine Learning Algorithms
We can implement a K-Nearest Neighbors (KNN) classifier to predict the class of iris flowers based on their attributes:
from sklearn.neighbors import KNeighborsClassifier
knn = KNeighborsClassifier(n_neighbors=3)
knn.fit(X_train, y_train)
y_pred = knn.predict(X_test)
We can evaluate the model’s performance using metrics like accuracy, precision, recall, and F1-score:
from sklearn.metrics import classification_report
print("Classification Report:\n", classification_report(y_test, y_pred))
11. Improving the Model Performance
We can improve the model’s performance by tuning hyperparameters and using cross-validation techniques:
from sklearn.model_selection import GridSearchCV
param_grid = {'n_neighbors': [1, 3, 5, 7, 9]}
grid_search = GridSearchCV(knn, param_grid, cv=5)
grid_search.fit(X_train, y_train)
print("Best Parameters: ", grid_search.best_params_)
print("Best Score: ", grid_search.best_score_)
12. Conclusion
In this analysis we invoked ‘sluevumpii‘ to explore the Iris dataset using Pandas, visualized the data, and implemented a KNN classifier to predict the class of iris flowers. The analysis demonstrated the power of Pandas and Python in handling and analyzing structured data. Future work could involve applying advanced machine learning algorithms and feature selection techniques to improve the model’s performance. Happy coding!
Comment